AI in Agriculture
The Future of Farming
The global population is expected to reach 10 billion by 2050, so the demand for food production is rapidly increasing. This growth puts immense pressure on agriculture to optimize yields, minimize resource consumption, and overcome various obstacles such as limited arable land, climate change, labor shortages, and decreasing soil fertility. Traditional farming methods are no longer sufficient to meet the rising food demands, leading to a surge in how Artificial Intelligence (AI) can transform Agriculture and Farming.
This research report explores the role of AI in agriculture, detailing its benefits, use cases, technological advancements, and challenges. It highlights AI’s potential to revolutionize farming by enabling data-driven decision-making, optimizing resource management, and automating labor-intensive tasks. Additionally, it reflects how the big names have successfully adopted AI in Agriculture and Farming, making a revolutionary impact.
Download the PDF
Current Challenges in Agriculture and Traditional Farming
Unpredictable Weather Patterns and Climate Change
Agriculture heavily depends on weather conditions, and climate change has made weather patterns increasingly unpredictable. Droughts, floods, and extreme temperatures can devastate crops, causing farmers severe economic losses. Farmers who depend on rainfall for irrigation experience crop failures during drought or when unexpected floods destroy fields.
Soil Degradation and Nutrient Depletion
Intensive farming practices, poor crop rotation, and excessive use of chemical fertilizers and pesticides have degraded soil health in many regions. As the soil loses its fertility, crop yields decline, making it easier to sustain large-scale farming with heavy inputs. Farmers are witnessing diminishing returns despite increasing their use of fertilizers as the soil's organic matter continues to decline.
Pest and Disease Outbreaks
Pests and diseases are constant threats to crop health. Traditional methods often rely on blanket pesticide application, which can be inefficient, costly, and harmful to the environment. Farmers may also struggle to detect infestations early, leading to significant losses. The invasion of pests like locusts has caused widespread crop devastation in several regions, affecting food security and livelihoods.
Inefficient Water Management
Water scarcity is one of the most critical challenges in agriculture. Traditional irrigation methods often waste water through inefficient distribution. In areas dependent on groundwater for irrigation, aquifers are depleting at an alarming rate due to over-extraction, threatening future farming viability.
Limited Access to Real-Time Data and Insights
Traditional farming often relies on manual methods and does not account for fast-changing variables like soil moisture, pest attacks, or upcoming weather conditions. Hence, it misses critical opportunities to intervene during the growing season, such as adjusting irrigation during heat waves or applying pest control early enough to prevent widespread damage.
Lack of Precision in Farming Practices
Fertilizer is applied uniformly, irrigation systems are operated manually, and crop treatments are the same for entire fields, regardless of varying needs. This leads to inefficient resource use and suboptimal results, as over-fertilizing some areas and under-fertilizing others leads to uneven crop growth and wasted inputs, reducing overall yield and increasing costs.
Modern Advancements in AI for Agriculture
The convergence of technologies such as AI, IoT, and Cloud Computing has enabled advanced data collection and processing capabilities, which drive advancements and innovations in agriculture, include:
AI-driven Predictive Analytics
Forecast weather conditions, crop yields, and disease outbreaks.
Robotics and Automation
Smart farming robots for planting, irrigation, and harvesting.
Real-time Monitoring Systems
Used for detecting disease, sorting harvested produce, and monitoring livestock of large agricultural fields.
Smart greenhouses
Automated systems that optimize conditions such as humidity, temperature, and light for plant growth.
Real-world Applications of AI in Agriculture and Farming
Smart Irrigation Systems
AI algorithms connected with IoT sensors provide real-time insights into soil moisture, weather conditions, and crop water requirements. AI can automatically adjust irrigation schedules and volumes, ensuring optimal water usage while promoting sustainability.
Deep Neural Network that integrates real-time weather, crop, and soil data to optimize irrigation with minimal human intervention. Combining these with Evapotranspiration Models and NDVI (Normalized Difference Vegetation Index) data from satellite imagery allows systems to optimize water usage at scale. In large irrigation networks, Decentralized Reinforcement Learning (DRL) models with multi-agent systems are implemented to account for regional variations in water availability.
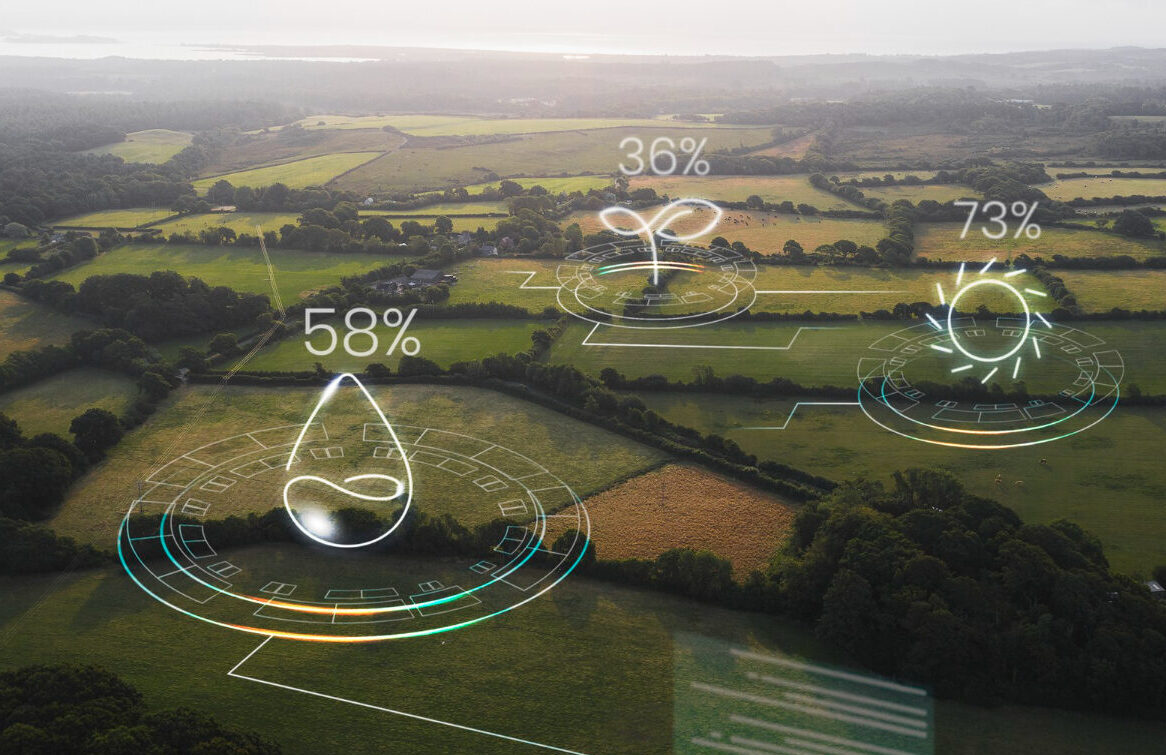
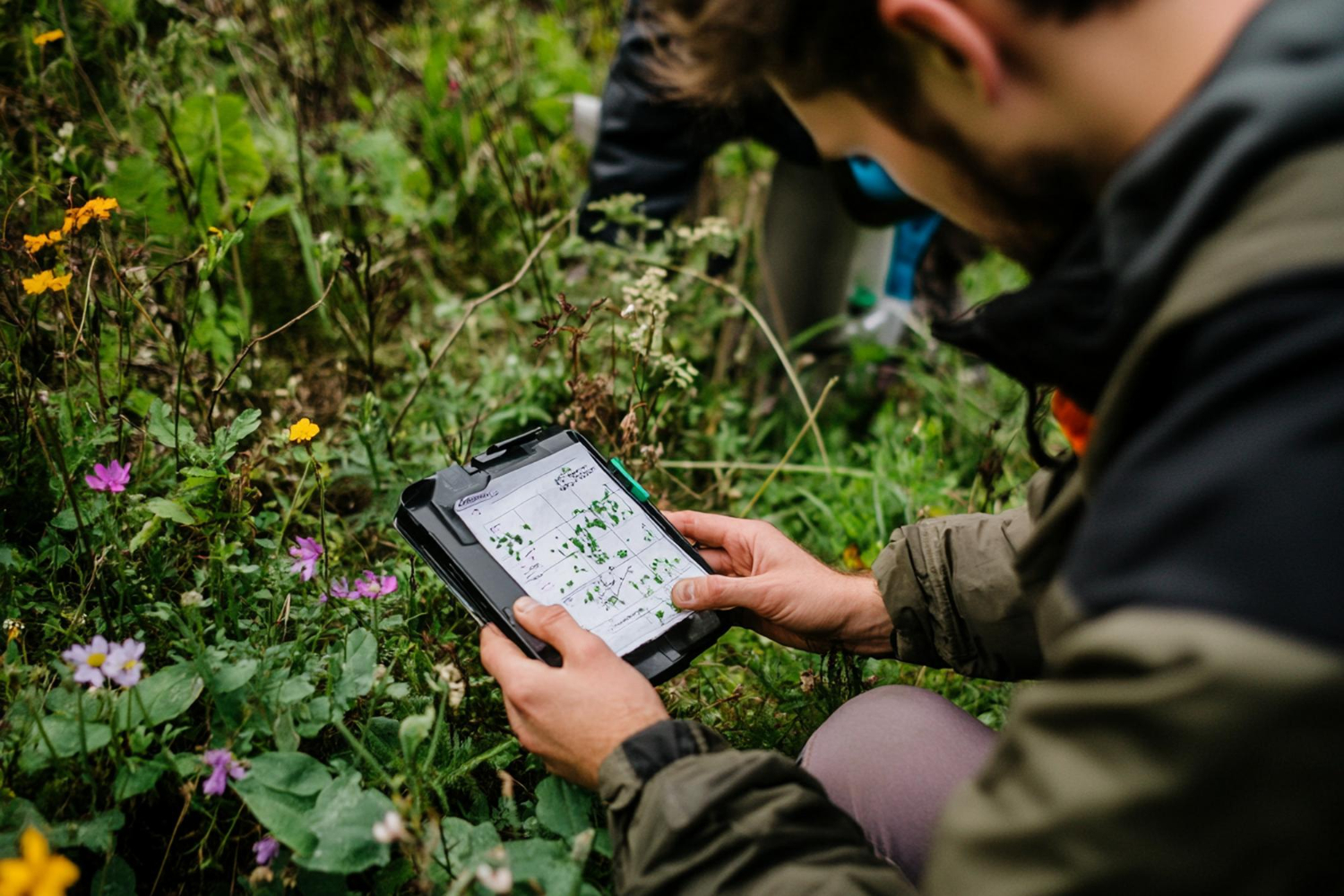
Crop Pest Detection and Health Monitoring
Computer vision algorithms and Deep Learning models such as PlantNet can assess soil nutrient levels and identify crop diseases or pests early, enabling farmers to take corrective actions promptly. For instance, AI has been used to detect apple black rot with over 90% accuracy.
By using Hyperspectral imaging or aerial imagery from drones and AI-based Spectral Angle Mapper (SAM) algorithms, farmers can monitor plant health down to the molecular level. Edge AI devices installed in the field can process images locally, reducing latency and enabling near-instant detection of pests or diseases like wheat rust or potato blight.
Predictive Weather and Climate Analytics
Hybrid Deep Learning Models, combining LSTM for time series data and Autoencoders for feature reduction, offer robust long-term climate forecasting. The latest advancements in Generative Adversarial Networks (GANs) can simulate different climate change scenarios and their impacts on farming regions, and Bayesian Inference helps assess the uncertainty in climate predictions.
Spatiotemporal GANs can generate high-resolution weather and climate prediction maps, improving forecasting accuracy for extreme weather events that may affect agricultural cycles. Multi-scale geospatial models predict spatial dependencies in weather conditions affecting regional crop productivity.
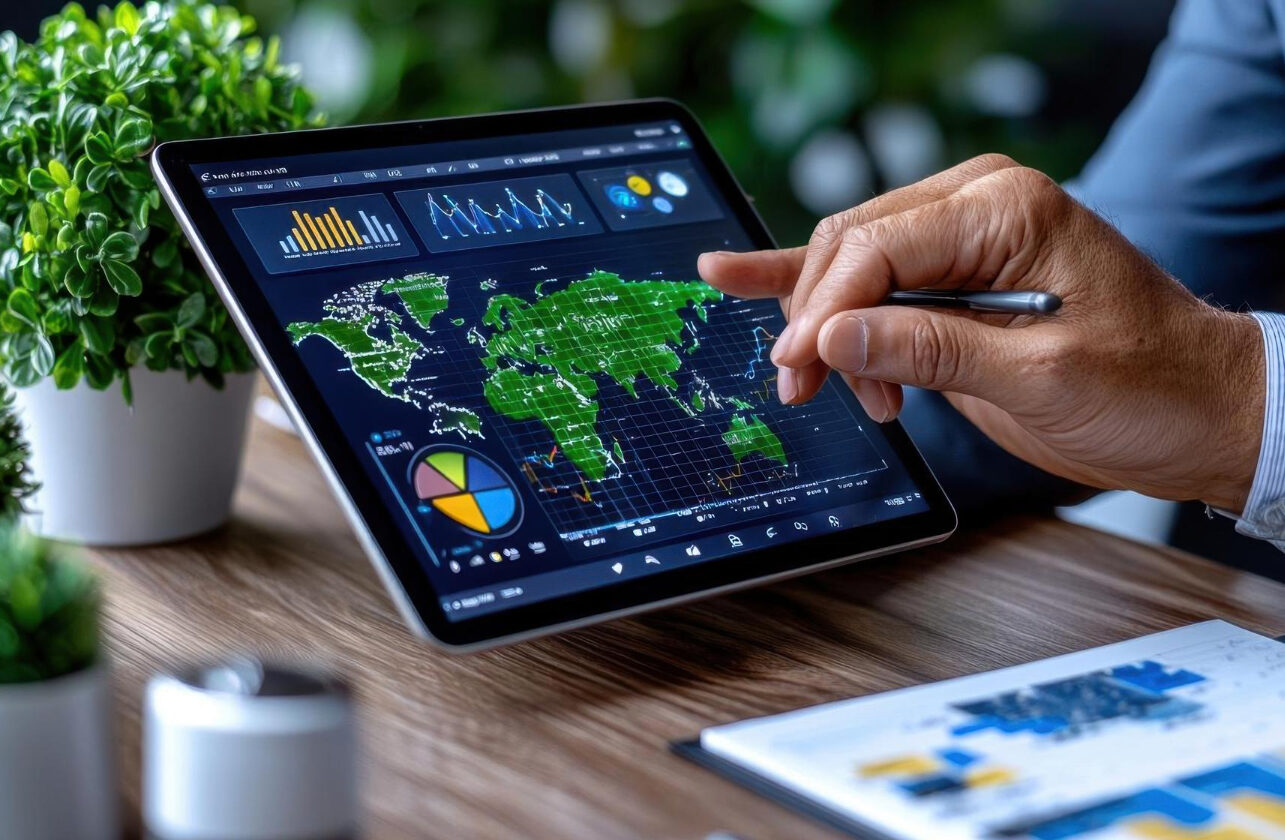
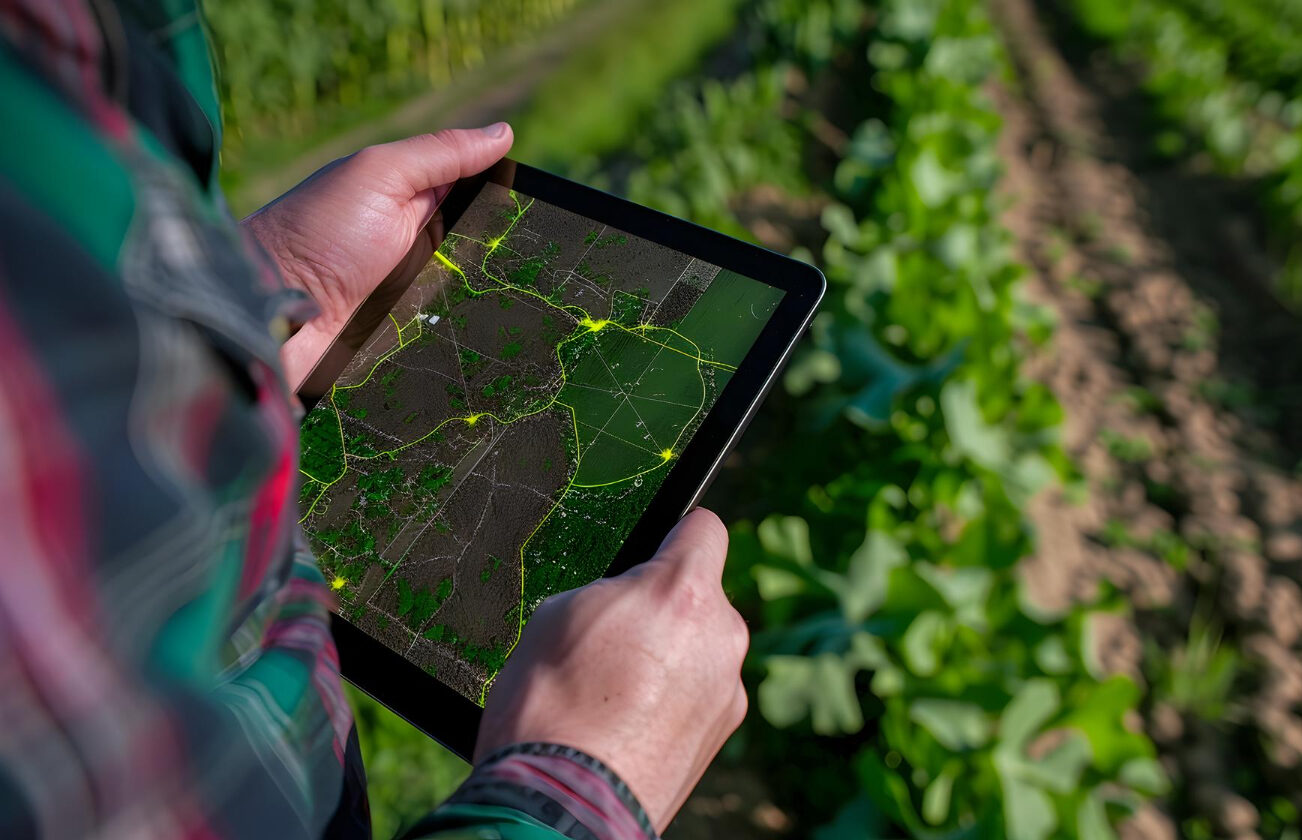
Yield Mapping and Prediction Models
Yield prediction models are critical for maximizing productivity and planning. Modern AI techniques such as Random Forests, XGBoost, and Deep Neural Networks (DNNs) offer high-accuracy yield forecasts based on extensive datasets that include weather conditions, soil data, historical crop yields, and satellite imagery.
CropNet yield prediction model integrates remote sensing data and time-series weather data with advanced LSTM models. Graph Yield Network utilizes GNN architecture to model the interdependencies between different geographic regions for more accurate large-scale yield forecasting. Furthermore, Federated Learning helps aggregate yield data from multiple sources, enabling geographically diverse yield prediction models.
Autonomous Weeding and Harvesting Equipment
AI-driven autonomous farming equipment is transforming traditional labor-intensive farming operations. Computer Vision and Reinforcement Learning models control robotic tasks such as automated weeding and harvesters that can navigate uneven terrain and optimize harvesting routes.
By applying Simultaneous Localization and Mapping (SLAM) algorithms, AI agents create accurate maps and navigate in large and uneven farm terrains without GPS reliance. Robots like AgBots employ deep learning models (e.g., YOLO or Faster R-CNN) for object detection and classification, identifying crops, weeds, and other objects to improve harvesting and planting accuracy.
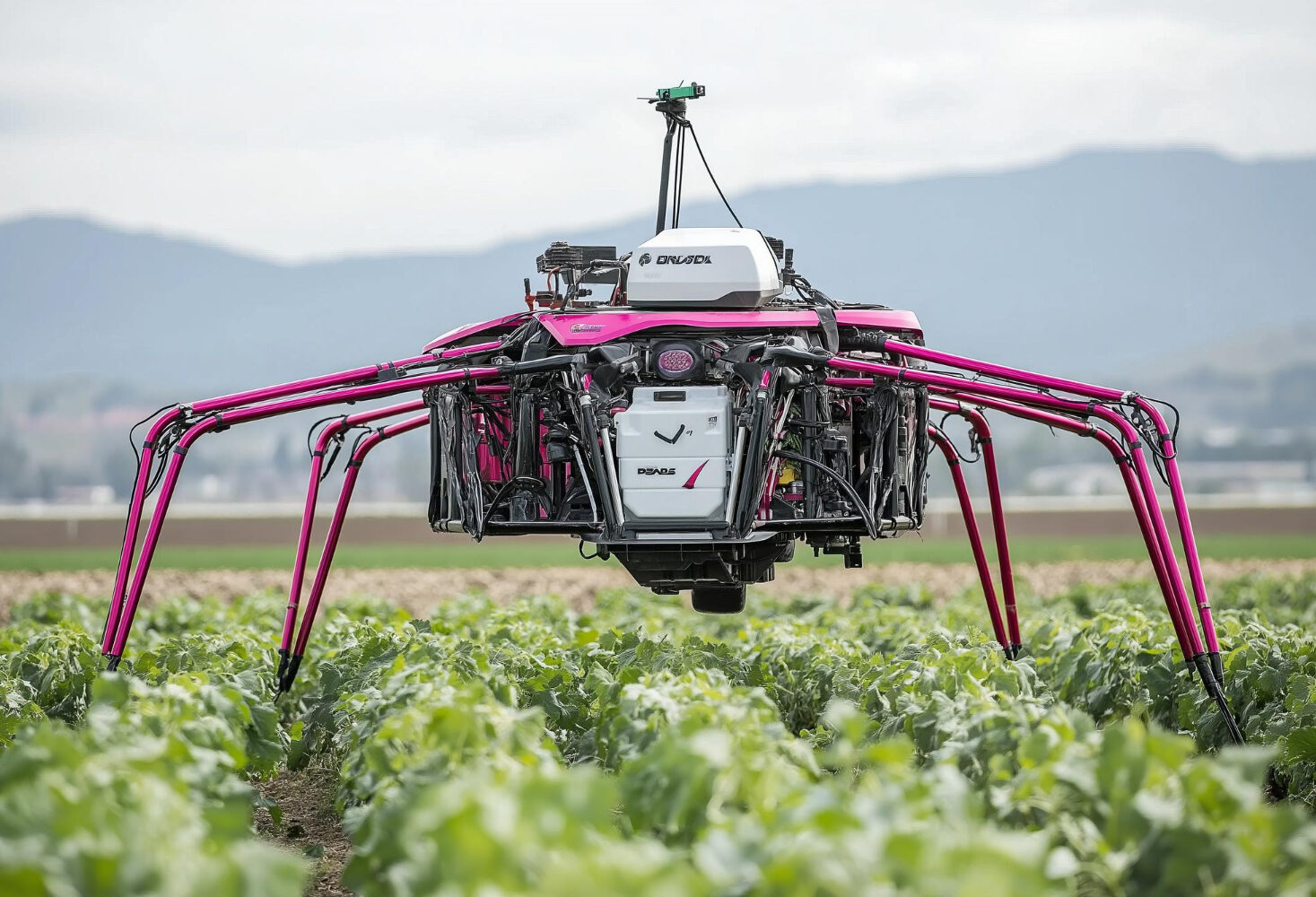
Benefits of Successful AI Adoption in Agriculture
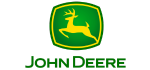
John Deere has implemented AI-powered Autonomous Tractors equipped with Computer Vision to plow fields more efficiently than traditional manual methods. These tractors can operate continuously without human intervention, reducing the time needed for large-scale farming operations.
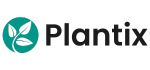
Plantix, an AI-driven app used by over 10 million farmers globally, leverages machine learning to identify plant diseases by analyzing images uploaded by farmers. The app provides early warnings and treatment suggestions, helping farmers protect their crops from potential diseases.
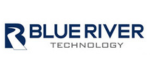
Blue River Technology developed an AI-based See & Spray system that uses Computer Vision to detect and target weeds in real-time. This system reduces the use of herbicides by up to 90%, making farming more sustainable and cost-effective.
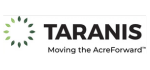
Taranis, an Israeli agri-tech company, uses AI and satellite imagery to predict crop yields for major commodity crops like wheat, corn, and soybeans. Their models help farmers make more informed decisions on crop rotations and harvest schedules, leading to more accurate yield predictions and reduced risk.
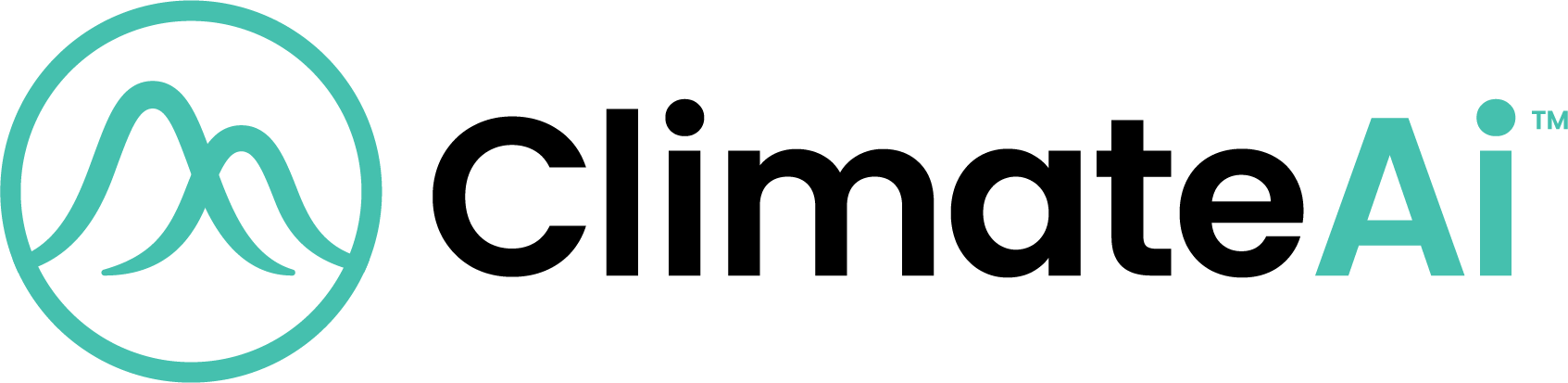
ClimateAi uses advanced machine learning models to predict climate risks for farmers months or even years in advance. Their platform helps farmers plan for future climate variability by providing actionable insights on adjusting crop schedules, planting locations, and resource allocation.
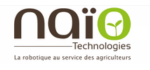
Naïo Technologies, a French robotics company, developed Dino, an autonomous weeding robot for vegetable farms. By replacing manual labor for weeding, Dino reduces labor costs significantly and allows farmers to focus on higher-value tasks.
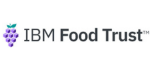
IBM Food Trust uses AI and blockchain technology to provide traceability across the entire agricultural supply chain, from farm to consumer. Ensuring transparency and real-time tracking of food products helps reduce spoilage, minimize supply chain disruptions, and build consumer trust.