AI has reshaped healthcare handling. Everything from spotting disease outbreaks to streamlining diagnostic imaging now involves the use of AI in one step or the other. AI’s ability to enhance accuracy, speed, and scale in patient care appears unquestionable. But as AI begins to hold a more central role in medical choices critical ethical and legal questions follow. Are we trading away patients’ rights to control decisions just to make systems run smoother? Are the data biases in these systems controlling clinical decisions? This blog explores the legal and moral challenges tied to AI in healthcare, diving into case laws, regulatory systems, and legal statutes.
AI in Healthcare: Its Potential and Promises
AI tools are making big changes in healthcare:
- Diagnostics: AI systems spot issues in radiology scans quicker than doctors can.
- Personalized Treatment: Machines analyze genetic data and create custom treatment plans.
- Predictive Analytics: Hospitals rely on AI to foresee worsening health problems or patient returns.
- Efficiency in Operations: AI handles scheduling, resource management, and lowers admin work.
These improvements lead to better accuracy earlier detection saving money, and care models that adapt to needs. This is vital where resources are limited.
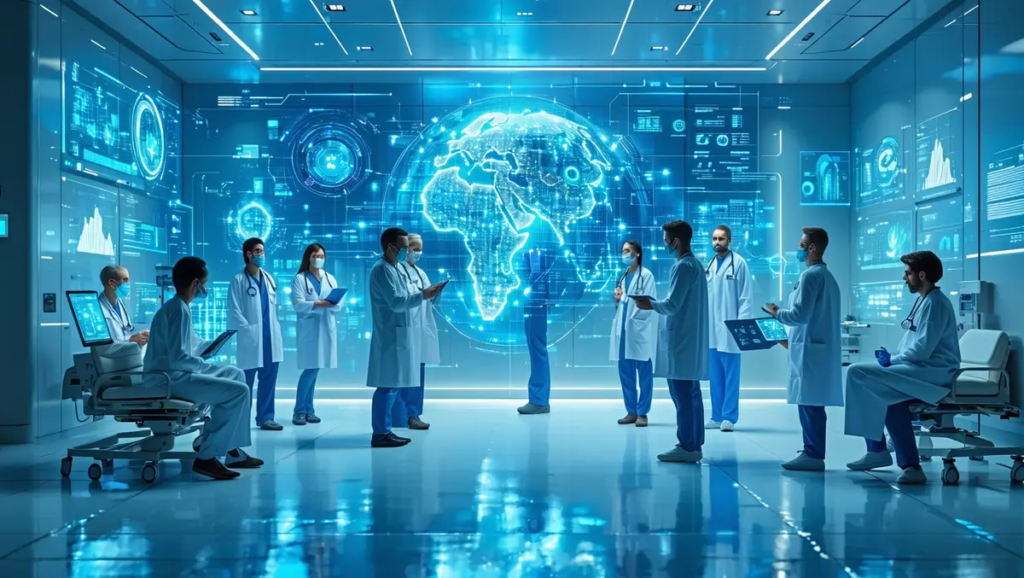
Rising Ethical Challenges
Even with these positives using AI in healthcare raises tough ethical and legal questions.
- Data Privacy and Consent: Big datasets power AI systems. Do patients understand how their information gets used? Are they aware when giving consent?
- Bias and Fairness: Training data can carry past biases. Some ethnic groups or genders might see worse outcomes with AI.
- Transparency and Explainability: Many advanced models act like “black boxes.” How can clinicians and patients rely on decisions they don’t understand?
- Autonomy and Human Oversight: Are doctors leaning too much on AI suggestions? Could this risk weakening their own judgment or limiting patient choices?
- Accountability and Liability: When AI in healthcare makes a mistake, who takes responsibility – the software creator, the hospital, or the doctor?
Legal systems are starting to address these problems, but a full agreement across countries is still developing.
Informed Consent and AI
The idea of informed consent relies on the patient knowing what their treatment involves. AI-based suggestions make this harder. How can you describe how an algorithm works to someone who isn’t familiar with it?
Related legal examples:
- Montgomery v Lanarkshire Health Board [2015] UKSC 11: This case in the UK changed how informed consent works. It states that doctors need to tell patients about all important risks tied to treatment options. In AI, this idea means revealing when algorithms are involved and explaining the risks linked to them.
- HIPAA (US) and GDPR (EU) set rules for explaining how personal data is handled. These laws also push for clearer guidelines to support informed consent when AI is involved.
This change calls for simpler educational resources to teach patients and demands that developers share more information.
Tackling Algorithmic Bias
Bias in AI models leads to unfair outcomes. For example, if an AI in healthcare system learns from Western data, it might fail at diagnosing illnesses in patients from non-Western regions. Possible ethical or legal fixes include:
Equal Protection Clause (14th Amendment US)1 and Article 14 of the Indian Constitution: These laws demand fairness and a ban on discrimination in services.
EU’s General Data Protection Regulation (GDPR)2 Article 22: Gives people the right to avoid significant decisions made by automated systems.
Building ethical AI should focus on these goals:
- Use datasets that cover different groups of people.
- Regular audits to check for inequality.
- Work with ethicists while designing systems.
Clear Communication without Oversimplifying
Understanding AI decisions is crucial in healthcare. Both patients and providers need to trust these systems, and that trust depends on knowing:
- The reasons for a specific diagnosis or suggestion.
- The importance AI assigned to various factors.
Legal frameworks like GDPR Recital 71 and the California Consumer Privacy Act (CCPA) ensure people can demand clarity about how automated decisions are made. Although interpretable AI in healthcare models are an option, they often compromise accuracy. Balancing model performance with interpretability remains a tough problem.
Human Oversight is Key
AI should support, not replace human judgment. Ethical use requires defining roles betweenhumans and AI.
Some recommendations include:
- AI can detect irregularities, but clinicians must make the ultimate call.
- Teams from different fields can assess AI results to decide whether to apply them.
- The FDA in the US and the MHRA in the UK recommend keeping human oversight in place when using high-risk AI tools.
This approach ensures responsibility stays with humans and keeps healthcare more personal.
Legal and Regulatory Overview
Rules are changing to manage how AI is used responsibly in healthcare.
- EU AI Act: Defines AI systems based on risk levels. It labels healthcare AI as “high-risk,” which demands clear documentation, transparency, and human involvement.
- US FDA: Oversees Software as a Medical Device, or SaMD. It has proposed a framework to handle updates in AI or ML-based software.
- India’s Draft Digital Personal Data Protection Act (2023)3: Lays out consent requirements and rights to control automated decision-making for data principals.
- Brazil’s Artificial Intelligence Bill (PL 21/2020)4: Establishes ethical guidelines and principles to guide how AI is managed.
- WHO Guidance on AI Ethics (2021)5: Lists six ethical rules to ensure AI works in health focusing on accountability and inclusion.
Case Law and Precedents
- T.J. Hooper (1932)6 (US): This case created a benchmark for technological standards of care. It matters today as AI becomes part of what is expected in medical practice.
- Schloendorff v. Society of New York Hospital (1914)7: This ruling introduced the idea of informed consent. It lays the groundwork for how to explain AI-based treatments to patients.
- United States v. Microsoft Corp. (2013)8: While not about medicine, this case on who controls data stored across borders affects how AI handles global data-sharing issues.
These cases, though not about AI, help answer new legal challenges surrounding AI and healthcare.
Future Directions and Ethical Design
Creating ethical AI means making ethics a part of every step in its development process:
- Ethics by Design: Include ethicists in designing the product.
- Participatory Methods: Build systems along with contributions from clinicians and patients.
- Constant Monitoring: Run audits and make updates after release to stop bias or drift.
Some guidelines that assist with this are:
- OECD AI Principles (2019)9
- UNESCO’s 2021 Ethics of AI Recommendation10
- Council of Europe’s focus on AI and Human Rights11
Strengthening Trust Using Compliance Tools
With regulations around AI changing, organizations require strong tools to handle AI compliance. At Adeptiv, we created an AI Compliance Tracker to assist teams in:
- Keeping track of global AI regulation alignment.
- Identifying potential ethical risks as they happen.
- Recording decisions .
Check out Adeptiv’s AI Compliance Software to address legal and ethical challenges while fostering trust in your AI solutions. Emerging discussions around the challenges and promises of AI in sensitive sectors like healthcare continue to gain attention — this article from The Economic Times explores how AI and healthcare remain complex, intertwined domains facing both innovation and regulation hurdles.
Conclusion
AI’s potential in healthcare is huge, but it needs a solid base of ethics and law. Understanding key issues like data privacy, fair algorithms, clear consent, and human oversight is a must. As AI in healthcare becomes central to medical care, we must make sure it helps rather than harms – even in subtle ways. Ethical AI goes beyond basic rules; it reflects kindness, responsibility, and the goal to harm none – even through technology. The future of healthcare will depend not on smarter machines but also on their fair and thoughtful use by society.