Artificial intelligence (AI) has become a cornerstone of modern social media platforms, shaping user experiences through personalized content, automated moderation, and targeted advertising. While AI enhances engagement and platform efficiency, its unchecked use raises ethical ai concerns regarding data privacy, algorithmic bias, and user rights. In particular, the regulation of AI in social media has gained significant attention in the United States and Europe, where policymakers are striving to balance innovation with user protection. As AI systems grow more sophisticated, implementing a comprehensive ai governance framework is crucial to safeguarding user data, promoting transparency, and fostering trust in digital interactions.
To achieve this, many organizations are turning to dedicated ai governance platforms to help monitor, audit, and align AI activities with ethical and legal standards. This article explores the role of AI in social media, the ethical challenges it presents, and the regulatory frameworks in the USA and Europe that seek to protect user rights.
The Role of AI in Social Media
AI plays a multifaceted role in shaping social media platforms, enhancing user experiences while addressing complex challenges. Some of the key aspects of AI’s use cases in social media applications include:
AI-Driven Content Moderation
AI systems revolutionize content moderation by efficiently processing vast amounts of user-generated content to identify harmful material such as hate speech, violence, or explicit imagery. Leveraging machine learning and natural language processing (NLP), these systems analyze text, images, and videos to detect violations of platform guidelines. Advanced features like semantic analysis and contextual understanding minimize false positives and ensure nuanced moderation. However, challenges persist in balancing automation with human oversight to address ambiguous cases and mitigate algorithmic biases. These challenges highlight the necessity of a structured ai governance framework to ensure moderation practices remain fair and unbiased.
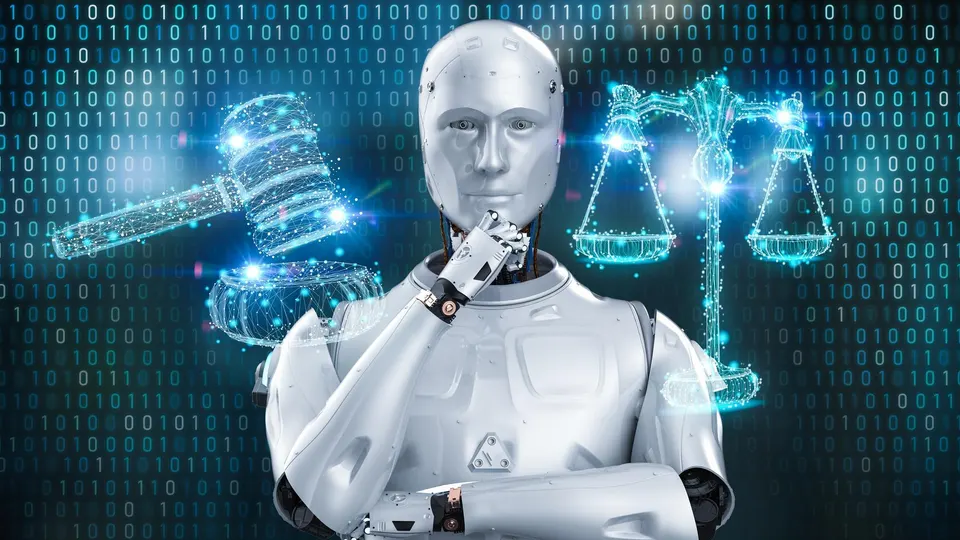
Algorithmic Recommendations and User Engagement
Recommendation algorithms powered by AI personalize user experiences by analyzing behavior patterns, preferences, and demographic data. These algorithms curate feeds, suggest connections, and promote content tailored to individual interests, driving engagement and retention. While effective in creating immersive experiences, they can inadvertently lead to echo chambers or amplify misinformation if not carefully managed. A robust ai governance platform can help monitor algorithmic decisions and ensure they align with ethical objectives and societal values.
AI in Personalized Advertising and Targeted Marketing
AI has transformed social media advertising through sophisticated targeting techniques. By analyzing extensive datasets on user behavior, preferences, and interactions, AI enables brands to deliver highly personalized ads that resonate with their audiences. This precision boosts ad performance but raises ethical concerns about privacy and the potential misuse of sensitive data. Deploying an ai governance platform supports transparent advertising practices and helps ensure compliance with privacy laws and user consent protocols.
Challenges of Misinformation and Deepfake Detection
AI is both a tool for combating misinformation and a contributor to its proliferation. Deepfake technology exemplifies this duality; while it enables realistic media manipulation that undermines trust, AI-powered detection systems analyze patterns, artifacts, and linguistic cues to identify manipulated content. Despite advancements in detection techniques like neural networks and forensic analysis, the evolving sophistication of deepfakes poses ongoing challenges. Establishing an adaptive ai governance framework is key to managing these risks effectively and maintaining information integrity.
The integration of AI into social media platforms underscores its transformative potential but also highlights the need for ethical structures such as ai governance platforms and frameworks to address the concerns discussed. These tools and standards not only provide oversight but also help promote a more responsible, fair, and transparent digital ecosystem.
Ethical Concerns in AI-Driven Social Media
Data Privacy and Unauthorized Data Collection
AI-driven social media platforms face significant ethical challenges, particularly regarding data privacy and unauthorized data collection. These systems often harvest vast amounts of user data—including biometric details, behavioral patterns, and emotional states – without explicit consent, raising risks of surveillance and identity theft. For example, AI algorithms analyze social media activity to build detailed profiles, enabling hyper-targeted advertising while exposing users to covert data-sharing practices that erode trust. The aggregation of seemingly innocuous data – such as location, timestamps, and interaction frequency—can infringe on privacy, akin to classified intelligence gathering in defense contexts. Regulatory efforts, supported by tools within an AI governance framework, such as the EU’s GDPR and California’s CCPA, aim to curb these practices, but enforcement remains challenging due to the global nature of social media platforms.
Algorithmic Bias and Discrimination
AI models trained on biased datasets perpetuate systemic inequalities. Algorithmic bias and discrimination compound ethical risks when skewed data and non-diverse design teams reinforce stereotypes and marginalize communities. Mitigation requires intentional dataset curation and fairness-aware models to ensure equitable outcomes. For instance, facial recognition tools have shown higher error rates for marginalized groups, while content moderation systems disproportionately flag posts using African American Vernacular English. These shortcomings highlight the need for a structured AI governance platform that can audit training data and enforce ethical AI principles.
AI systems also inadvertently amplify misinformation due to biased training, emotional manipulation, algorithmic virality, adversarial attacks, and technical gaps. Misinformation becomes particularly persuasive when AI-generated, thanks to emotionally charged language and vivid narrative structures. Social media algorithms – designed to prioritize engagement—further promote misleading content. The “liar’s dividend” erodes trust in all online information, blurring the line between truth and deception. Adversarial actors manipulate AI models to bypass safeguards, and the detection tools – like deepfake detectors—struggle to keep pace, especially in non-English contexts. A robust AI governance framework is essential for setting ethical boundaries, enhancing transparency, and deploying standardized detection tools globally.
Transparency and Explainability in AI Decisions
The opaque or “black box” nature of AI undermines accountability, especially on social media. Users and regulators often have no visibility into how content is prioritized or moderated. Platforms rarely disclose how recommendation engines elevate viral content over verified information, contributing to misinformation spread. Singapore’s Model AI Governance Framework emphasizes explainability as a core value, pushing platforms to clarify decision-making processes. However, neural networks’ complexity poses technical hurdles, making tools like semantic ontologies essential to interpret algorithmic logic. Embedding transparency requirements into an AI governance platform can support explainability, auditability, and user trust.
Psychological Impact of AI-Driven Engagement Strategies
AI-driven engagement strategies – such as dopamine-triggering notifications and algorithmically amplified content – fuel addiction, anxiety, and social comparison, especially among teenagers. These algorithms exploit human psychology to maximize user retention, often at the cost of mental well-being. A notable example is Microsoft’s “Tay” chatbot, which adopted offensive language based on user interactions. Ethical design principles, including “privacy by default” and user-centric transparency dashboards, should be core components of any modern AI governance platform, aligning AI goals with public health and societal welfare.
Mitigation Strategies and Governance
According to IEEE research, embedding ethical principles—like fairness and transparency—into AI during training, operation, and reinforcement stages is vital. Continuous human oversight, ethics boards, and simulated test environments can validate AI decisions before deployment. These approaches ensure AI evolves within acceptable moral and societal boundaries. An effective AI governance framework must support these strategies through clear policies, shared standards, and oversight tools to prevent misuse. A well-structured AI governance platform facilitates this by offering mechanisms for ethical compliance, real-time auditing, and stakeholder participation across AI’s lifecycle.
Regulatory Frameworks in the USA and Europe
USA: Federal and State-Level Data Protection Laws
The United States lacks a comprehensive federal privacy law, instead relying on a fragmented landscape of state and sector-specific regulations. Federal laws like the FTC Act, HIPAA, GLBA, and COPPA provide some oversight, but are limited in scope. States such as California have taken the lead with the CCPA and CPRA, expanding consumer rights, limiting data usage, and introducing independent enforcement bodies like the CPPA. Other states, including Colorado and Virginia, have introduced similar laws that stress transparency and consumer control.
However, businesses operating nationwide must navigate a complex regulatory patchwork, leading to inconsistent privacy protections. Enforcement difficulties further emphasize the need for a unified national policy and a scalable AI governance platform that simplifies compliance while protecting user rights. Tools like these can streamline ethical practices across jurisdictions and prevent regulatory fragmentation from undermining consumer protections.
Europe: GDPR and AI Act Implications for Social Media
Europe has established a robust AI governance framework to tackle the ethical and legal challenges posed by AI in social media, with the General Data Protection Regulation (GDPR) and the Artificial Intelligence Act (AI Act) playing central roles.
GDPR protects personal data and privacy across all EU member states, requiring platforms to obtain explicit user consent for data collection and processing. It also limits automated decision-making and profiling, requiring platforms to inform users and provide opt-out options. Non-compliance can result in severe penalties, up to €20 million or 4% of global annual revenue.
The EU AI Act, set to fully enforce key provisions from February 2025, complements GDPR by addressing the use of AI systems in social media. It adopts a risk-based approach, categorizing AI systems into unacceptable, high, limited, and minimal risk. High-risk systems, such as social media algorithms used for content recommendations or targeted advertising, fall under high-risk systems due to their potential impact on privacy and freedom of expression. The AI Act mandates transparency in AI-generated or modified content, prohibits systems that manipulate vulnerable groups, and bans social scoring practices that foster discrimination or exclusion.
Comparison of Enforcement Mechanisms
United States: Fragmented and Decentralized Enforcement
In the U.S., enforcement of privacy and AI-related laws is fragmented across federal and state levels. Federal agencies like the Federal Trade Commission (FTC) play a central role in regulating unfair or deceptive practices, including data misuse, but their authority is limited to specific cases rather than systemic oversight. Sector-specific laws such as HIPAA (healthcare) or COPPA (children’s online privacy) add another layer of complexity, with enforcement divided among various federal agencies. At the state level, laws like California’s CCPA/CPRA are enforced by the newly established California Privacy Protection Agency (CPPA) and state attorneys general. However, this decentralized approach creates inconsistencies in enforcement across states, making compliance challenging for companies operating nationwide. Penalties under U.S. laws are generally lower compared to Europe, with fines often capped at modest amounts per violation.
Europe: Centralized and Uniform Enforcement
In contrast, Europe employs a centralized enforcement model under the GDPR and the upcoming AI Act. Each EU member state has a designated Data Protection Authority (DPA) responsible for enforcing GDPR locally, while the European Data Protection Board (EDPB) ensures coordination and consistency across the bloc. GDPR imposes stringent penalties for non-compliance—up to €20 million or 4% of global annual revenue—making it one of the most rigorous frameworks globally. The AI Act introduces additional oversight for high-risk AI systems, requiring platforms to conduct risk assessments and register their systems in an EU-wide database. Violations under the AI Act can result in fines up to €35 million or 7% of annual turnover, emphasizing the strength of Europe’s AI governance platform.
Key Differences
- Scope and Uniformity: Europe’s GDPR applies uniformly across all member states, ensuring consistent enforcement, whereas U.S. laws vary widely by state and sector.
- Penalties: European penalties are significantly higher and designed to deter violations, while U.S. fines are comparatively lenient.
- Oversight Bodies: Europe relies on centralized bodies like DPAs and the EDPB for enforcement, whereas the U.S. divides responsibilities among multiple federal and state agencies.
- Focus Areas: While GDPR emphasizes user consent and data minimization, U.S. laws like CCPA focus more on transparency and opt-out rights.
Global Initiatives and Sector-Specific Challenges
Global Initiatives: Establishing Ethical AI Standards
International organizations have taken significant steps to create a universal AI governance framework. UNESCO’s Recommendation on the Ethics of Artificial Intelligence emphasizes transparency, accountability, and privacy while banning invasive practices like social scoring and mass surveillance. It also promotes AI’s potential for inclusivity, environmental sustainability, and disaster management. Similarly, the OECD’s updated AI Principles advocate for trustworthy AI that respects human rights and democratic values, focusing on inclusive growth and sustainable development. These global efforts aim to harmonize AI regulations across borders, providing policymakers with tools to assess AI’s societal impact and ensure its deployment aligns with ethical norms. However, geopolitical tensions between major AI players like the U.S. and China often hinder cross-border consensus, complicating the establishment of universally accepted standards.
Sector-Specific Challenges in Social Media
AI regulations vary significantly across industries, presenting unique challenges for social media platforms. In advertising, AI-powered tools must comply with strict privacy laws such as GDPR’s consent requirements and the EU AI Act’s transparency mandates. For example, targeted advertising algorithms are classified as high-risk systems under the AI Act due to their potential to manipulate user behavior. In content moderation, platforms face obligations to disclose how algorithms detect harmful material while addressing biases that could lead to unfair censorship or discrimination. Additionally, copyright laws struggle to accommodate AI-generated content, raising legal uncertainties about ownership in generative AI applications. These challenges call for a specialized AI governance platform that addresses the nuances of the social media landscape.
Enforcement Challenges
Challenges in Multinational Compliance
For multinational companies operating in both regions, reconciling these regulatory differences is a major challenge. For instance, GDPR’s strict opt-in consent requirements often conflict with CCPA’s opt-out model. Additionally, cross-border data transfers between the EU and U.S. remain contentious due to differing privacy standards, as seen in the invalidation of mechanisms like Privacy Shield.
By comparison, Europe’s centralized approach offers greater clarity but imposes stricter compliance burdens, while the U.S.’s fragmented system provides flexibility but creates regulatory uncertainty. These differences underscore the need for harmonized AI governance frameworks to streamline compliance for businesses operating across jurisdictions.
Best Practices for Ethical AI in Social Media
Adopting ethical AI practices in social media requires a multifaceted approach to balance innovation with user protection. Privacy-preserving AI techniques, such as differential privacy and federated learning, minimize data exposure by anonymizing datasets and decentralizing model training, ensuring sensitive user information remains secure.
Differential Privacy: This technique introduces mathematically calibrated noise into data analysis processes, ensuring that individual data points cannot be identified or inferred from aggregate results. It’s widely used in applications like census data analysis and behavioral tracking, offering provable guarantees against re-identification attacks.
Federated Learning: Unlike traditional centralized machine learning, federated learning trains models across decentralized devices or nodes without transferring raw data to a central server. This method is particularly effective for mobile and IoT applications, preserving user privacy while enabling model improvement.
Transparent AI models and user explainability are crucial for building trust, particularly in high-stakes domains like healthcare, finance, and social media moderation. Explainable AI (XAI) uses techniques such as feature importance analysis and model visualization to make complex algorithms more understandable.
Fairness and bias mitigation strategies demand proactive measures, including diversifying training datasets and deploying fairness-aware algorithms. Research from the U.S. Army has shown that AI systems can internalize harmful narratives from social media, emphasizing the need for continuous audits and bias correction.
Responsible AI governance requires strong oversight mechanisms, including third-party audits and ethics boards. A proposed three-phase training approach – training, operation, and reinforcement – advocates for continuous human oversight before granting AI systems increasing autonomy. Platforms should also adopt “privacy by design” principles, integrating ethical guidelines during system development rather than after deployment
For those exploring responsible innovation and ethical tech practices, Ball State University Libraries has launched a research guide on ethical AI use, offering a curated resource for students, researchers, and institutions alike..
Conclusion
The integration of artificial intelligence (AI) into social media has revolutionized how we connect, communicate, and consume content, but it also presents profound ethical challenges. From data privacy concerns and algorithmic biases to the amplification of misinformation, the unchecked use of AI risks undermining trust and societal well-being. As highlighted in the research document and corroborated by global trends, addressing these challenges requires a robust ethical foundation guided by a comprehensive AI governance framework that prioritizes transparency, accountability, and fairness.
Regulatory efforts like the EU’s GDPR and AI Act, alongside U.S. state-level laws such as CCPA/CPRA, demonstrate the growing commitment to safeguarding user rights while fostering innovation. At the same time, the adoption of a well-defined ethical AI governance framework ensures that organizations implement ethical guardrails from the design phase through deployment. Emerging trends point toward a future where decentralized social media platforms powered by blockchain offer alternative models for ethical data management, while AI tools enhance accessibility for users with disabilities.
Moreover, the rise of advanced ethical AI governance platforms is enabling real-time monitoring and enforcement of ethical standards. These platforms empower regulators and developers alike to track compliance, conduct audits, and respond to emerging risks effectively. However, as noted in both the research document and recent studies, the success of these initiatives depends on collaborative efforts involving governments, tech companies, and civil society. Stakeholder engagement, third-party audits, and iterative feedback loops are essential to ensuring that AI systems evolve responsibly.
Ultimately, embedding ethics into AI systems through human-curated training and ongoing oversight can pave the way for responsible AI autonomy. By aligning technological advancements with societal values and by leveraging collective human knowledge within a strong and the best AI governance platform, social media platforms can foster trust, inclusivity, and accountability in digital spaces. We can harness AI’s transformative potential while mitigating its risks. The time to act is now – through proactive governance and ethical innovation, we can ensure that AI serves as a force for good in shaping the future of social media and beyond.